LSF tools and corpora
Since the creation of automatic processing softwares dedicated to sign languages (SL) is a long-term task, we have launched in parallel three complementary studies that have focused on the creation of tools around SL corpora.
1. Computer Aided Translation Software French/French Sign Language
Machine translation requires aligned data and very few exist in French/French Sign Language. One way to build more is to develop concordancer-type tools in a computer-assisted translation (CAT) environment, which should allow for the collection of alignments made by professional translators. This type of software does not yet exist for SL, so we have launched a study (Marion Kaczmarek’s thesis) on this subject.
In order to determine more specifically the stages of French/French Sign Language translation, two studies were carried out with professionals in the field: on the one hand, brainstorming to get them to reflect and verbalize their needs and everyday problems in their professional practices, and on the other hand, sessions where pairs of translators were filmed while they worked on the translation of journalistic texts. This allowed to draw up a list of the tasks inherent in SL translation, but also to determine whether they were systematic and ordered.
Based on these observations, a list of features was developed for a CAT software: source text processing (e.g. automatic identification of dates and named entities), possibility to change sequence order, since this order is not identical in SL (which always places context before) and in spoken languages, presence of help modules (lexicon, encyclopedia, geographical map, etc.), translation memory and prompter for the final realization.
Two prototypes were made. An interface that implements the principles set out in the specification (Figure 1), as well as a bilingual concordancer (Figure 2), based on a parallel body of texts and video translations in French Sign Language. It is currently accessible online on a dedicated platform, and allows testers to make word or word set queries so that they can view video clips in French Sign Language in context. It remains to be integrated into the CAT software.
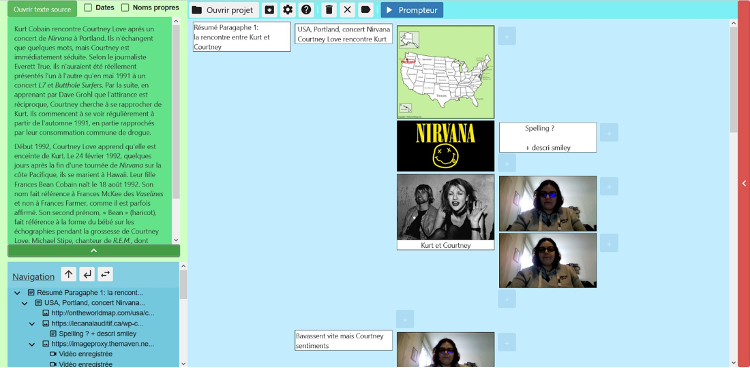
Figure 1: Text to LSF CAT software prototype interface.
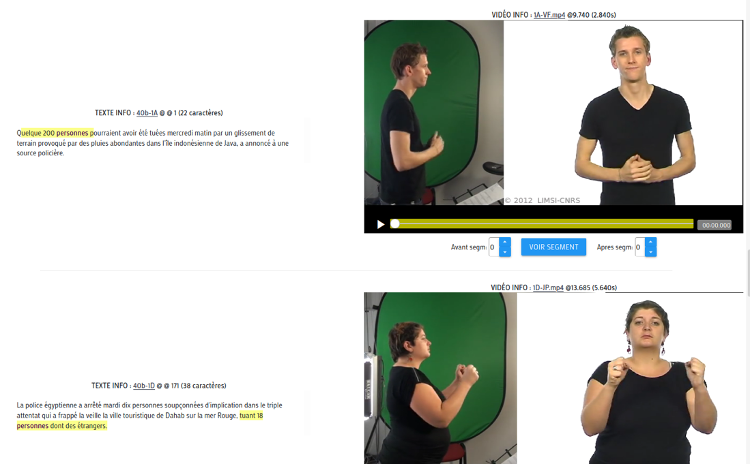
Figure 2: Text/LSF online concordancer
Related publications:
- Kaczmarek, M. Filhol (2019), Computer-assisted Sign Language translation: a study of translators’ practice to specify CAT software, 6th International Workshop on Sign Language Translation and Avatar Technology (SLTAT 2019), Hamburg, Germany.
- Kaczmarek, M. Filhol (2019), Assisting Sign Language Translation: what interface given the lack of written form and the spatial grammar?, 41st Translating and the Computer (TC 2019), Londres, United Kingdom, 83-92.
- Kaczmarek, M. Filhol (2020), Use cases for a Sign Language Concordancer, 9th Workshop on the Representation and Processing of Sign Languages (WRPSL@LREC 2020), 12th Int Conf on Language Resources and Evaluation (LREC2020), Marseille, France, 113-116.
- Kaczmarek, M. Filhol (2020), Alignments Database for a Sign Language Concordancer, 12th Int Conf on Language Resources and Evaluation (LREC2020), Marseille, France, 6069-6072.
- Kaczmarek, A. Larroque, M. Filhol. Logiciel de Traduction Assistée par Ordinateur des Langues des Signes. Colloque Handiversité 2021, Apr 2021, Orsay, France.
- Kaczmarek, A. Larroque (2021), Traduction Assistée par Ordinateur des Langues des Signes : élaboration d’un premier prototype, 28ème conférence sur le Traitement Automatique des Langues Naturelles, 2021, Lille, France. pp.108-122.
- Kaczmarek, M. Filhol. Computer-assisted sign language translation: a study of translators’ practice to specify CAT software – 2021 version. Machine Translation, Springer Verlag, 2021, 35 (3), pp.305-322.
2. Automatic subtitle/LSF alignment
The second study explored whether such alignments can be achieved automatically. One study (Hannah Bull’s thesis) was devoted to the automatic alignment between subtitles and LSF segments with a machine learning approach. To do this, we developed a parallel corpus of nearly 30 hours, based on the website of Media’Pi!, already a partner of the project for the constitution of the Rosetta corpus (see Noémie Churlet testimony). A first version of the system was based on prosodic elements such as pauses in motion, or specific postures. The current work is to identify segments in the video that correspond to “subtitling units,” using a machine learning approach illustrated in Figure 3.
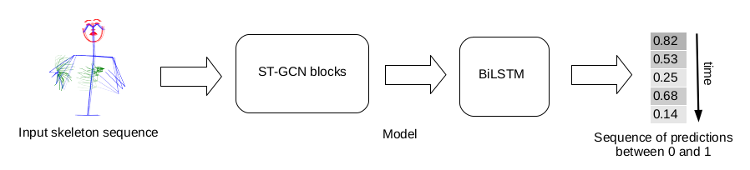
Figure 3: Architecture of the system for segmenting video of French Sign Language into units of type sentences.
Related publications:
- Bull, A. Braffort, M. Gouiffès (2020), MEDIAPI-SKEL – A 2D-Skeleton Video Database of French Sign Language With Aligned French Subtitles, 12th Int Conf on Language Resources and Evaluation (LREC2020), Marseille, France, 6063-6068.
- Bull, A. Braffort, M. Gouiffès (2020), Corpus Mediapi-skel sur Ortolang : https://www.ortolang.fr/market/corpora/mediapi-skel (56 téléchargements)
- Bull, A. Braffort, M. Gouiffès (2020), Automatic Segmentation of Sign Language into Subtitle-Units, Sign Language Recognition, Translation & Production workshop (SLRTP20), 16th European conference on computer vision (ECCV’20) – Best paper award.
3. Anonymization of animations
Finally, a third study (Félix Bigand’s thesis) focused on the nature of the data collected in the project. Since the generation of animations is done from data captured using a very precise motion capture system, it is quite possible to recognize the person who has been “mocapped,” even if the features of the avatar are very different from those of the recorded person, which can be problematic in some contexts. The objective is to identify which parameters of the movement define the individual style of the signers, so that they can play on these parameters to make the recorded SL anonymous, while keeping it perfectly understandable.
After studying the complex structure of movements in French Sign Language, work focused on the problem of identifying speakers by their movement, with 3 other contributions:
- A visual perception study that demonstrated that subjects were able to recognize the person who was speaking from a simple short extract as moving light spots (Figure 4).
- A machine learning model based on the statistical data from the MOCAP1 corpus for each signer (Figure 5). The kinematic signature parameters of the identity of the signers could be defined using this model.
- A synthesis algorithm that allows the parameters defining the kinematic signature of the signers to be modified, which can be applied to anonymization (FIG. 6).
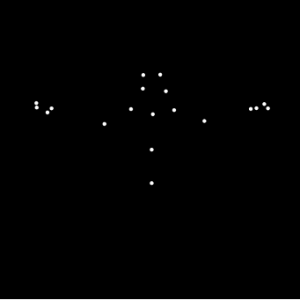
Figure 4: An example of light dot display used in the experiment.
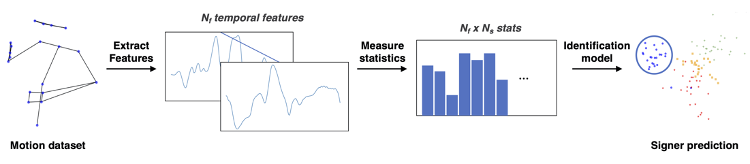
Figure 5: A diagram of the steps used in the machine learning identification model.
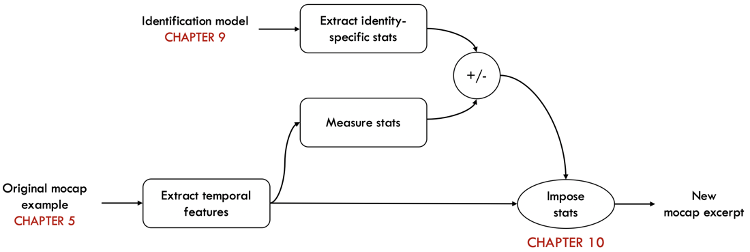
Figure 6: Diagram of the steps of the synthesis algorithm for kinematic identity control.
Related publications:
- Bigand, A. Braffort, E. Prigent, B. Berret (2019), Signing Avatar Motion: Combining Naturality and Anonymity, 6th International Workshop on Sign Language Translation and Avatar Technology (SLTAT 2019), Hambourg, Germany.
- Bigand, E. Prigent, A. Braffort (2019), Animating virtual signers: the issue of gestural anonymization, 19th International Conference on Intelligent Virtual Agents (IVA 2019), Paris, France.
- Bigand, E. Prigent, A. Braffort (2019), Retrieving Human Traits from Gesture in Sign Language: The Example of Gestural Identity, 6th International Symposium on Movement and Computing (MOCO 2019), Tempe, United States.
- Bigand, E. Prigent, A. Braffort, B. Berret (2019), Animer un signeur virtuel par mocap : le problème de l’identité gestuelle, 5è Journée de la Fédération Demeny-Vaucanson (FéDeV 2019), Palaiseau, France.
- Bigand, E. Prigent, A. Braffort (2020), Person Identification Based On Sign Language Motion: Insights From Human Perception And Computational Modeling, 7th International Conference on Movement and Computing (MOCO’20).
- Bigand, E. Prigent, B. Berret, A. Braffort. How Fast Is Sign Language? A Reevaluation of the Kinematic Bandwidth Using Motion Capture. 29th European Signal Processing Conference (EUSIPCO 2021), 2021.
- Bigand, E. Prigent, B. Berret, A. Braffort. Machine Learning of Motion Statistics Reveals the Kinematic Signature of the Identity of a Person in Sign Language. Frontiers in Bioengineering and Biotechnology, Frontiers, 2021, 9.
- Bigand, E. Prigent, B. Berret, A. Braffort. Decomposing spontaneous sign language into elementary movements: A principal component analysis-based approach. PLoS ONE, Public Library of Science, 2021, 16 (10).